No matter how much training, skill, motivation, or experience, humans are compromised by reduced attention or impaired alertness. Depression, stress, fatigue, and burnout are all consequences of unhealthy, and unsafe work conditions. Our research focuses on the population of desk-bound employees, who are typically expected to sit in one space for periods of two hours, and more while performing a specific activity or sequence of tasks. For those who work predominantly using computers, there is growing scope to augment task performance using artificial virtual agents.
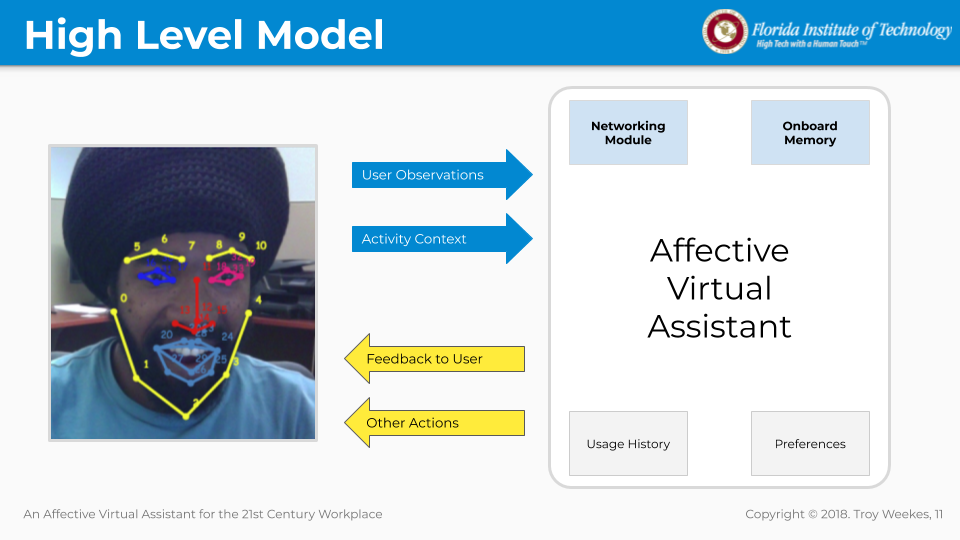
We propose AVA, an interactive smart assistant, that is designed to playfully augment user performance in the workplace without the user having to say anything. Universally, humans express emotions and feelings through their faces (Ekman, 1997). Our smart assistant observes a human user, infers emotional state, and reasons to effect relevant feedback. The assistant embodies an affective model of the subject that is used for advising more effective actions, behaviors, and work practices.
A system of productions was formulated from expected features that were found in the video streams, which represent the emotional state. The Markov Decision Process (MDP) is defined as a tuple (B, A, τ, r, γ) modeled in discrete-time and that the agent was unable to directly observe the underlying human emotional state (only expressions of emotion). Therefore, the model was required to maintain a probability distribution over the set of possible states, based on a set of observations, observation probabilities, and the underlying MDP.
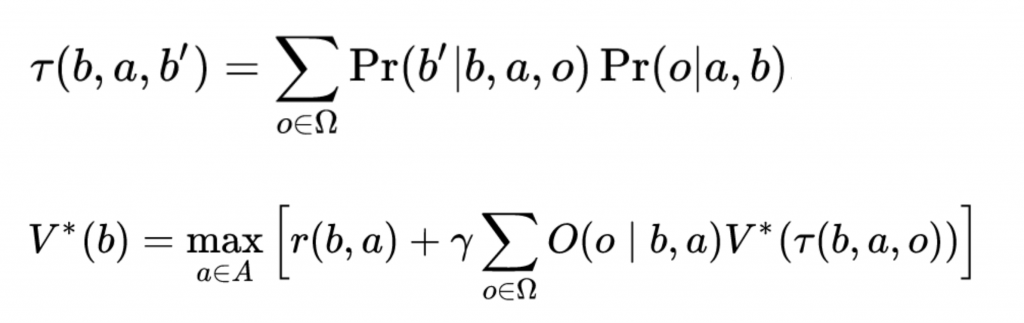
We implemented a real-time facial expression recognition algorithm. The live video streams are processed as discrete frames with 34 AUs per face. AVA detects and extracts facial features according to the Facial Action Coding System (FACS). Classified emotions are used to generate intelligent actions, advice, and prompts. AVA integrates facial expression with objective EEG & eye-tracking data. In this research project, we simulated and tested a human-in-the-loop model within the context of a real-world joint-cognitive system.
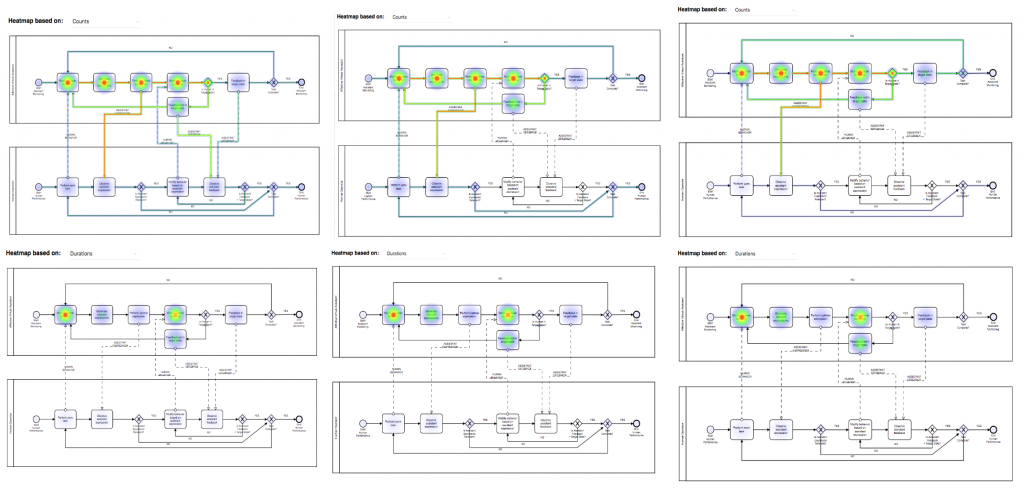
We plan to integrate our facial expression model with objective measures of physiological arousal, brain activity, and eye-tracking to generate a comprehensive model of behavior in the office workplace.